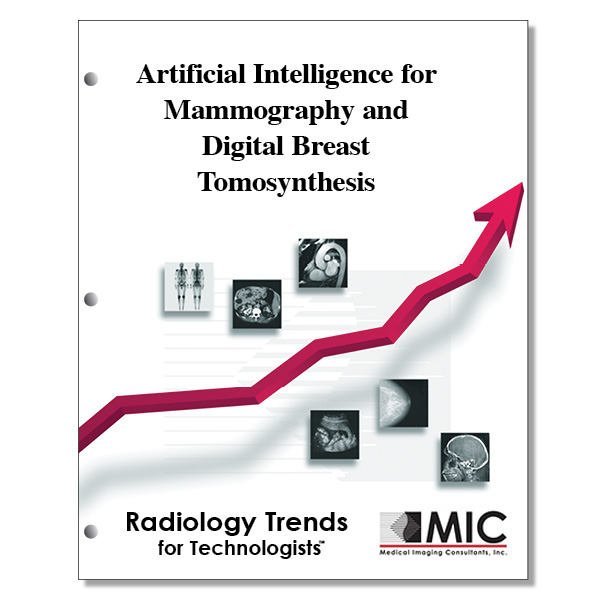
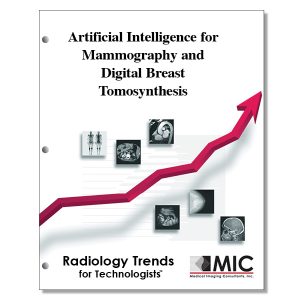
Artificial Intelligence for Mammography and Digital Breast Tomosynthesis
The potential of deep learning techniques for mammography and DBT are presented, and approaches to improve upon available CAD systems for mammography are discussed.
Course ID: Q00638 Category: Radiology Trends for Technologists Modality: Mammography2.5 |
Satisfaction Guarantee |
$29.00
- Targeted CE
- Outline
- Objectives
Targeted CE per ARRT’s Discipline, Category, and Subcategory classification:
[Note: Discipline-specific Targeted CE credits may be less than the total Category A credits approved for this course.]
Mammography: 1.00
Image Production: 1.00
Image Acquisition and Quality Assurance: 1.00
Outline
- Introduction
- Conventional AI
- Why Deep Learning
- How Deep Learning Works in Mammography
- Technical Challenges Unique to Mammography
- Clinical Applications
- Increase the Cancer Detection Rate and Reduce the Recall Rate
- Quantitative and Reproducible Assessment of Breast Density to Stratify Risk for Breast Cancer
- Applying Deep Learning Algorithms to DBT
- Radiomics
- Future Directions
- Conclusion
Objectives
Upon completion of this course, students will:
- understand the reading time for DBT compared to that for FFDM
- recognize the different meanings of CAD
- learn the type of deep neural network used on most medical image AI
- know the term that means mimicking human intelligence by learning from data and by applying manually defined decision rules
- realize the significance of the AI DREAM challenge
- learn the challenges of applying a CNN to mammography data
- recognize how an AI system can relay its predictions to humans
- understand reimbursement rules for CAD
- discover how AI can be used to reduce radiologist’s reading time
- realize why it’s important to identify if a patient has dense breast tissue
- know how different manufacturers’ DBT systems differ
- learn how AI cancer detection systems for DBT were trained
- recognize how AI can improve limited sensitivity of DBT in dense breast tissue
- learn what happens if synthetic mammograms are generated from tomosynthesis data using deep learning techniques
- know the goal of quantitative radiomics
- identify AI studies that rely on details of extracted breast features
- contemplate the sources consulted by a radiologist when evaluating a breast exam
- define precision medicine
- list the roles AI is likely to play in mammography
- list shortcomings of AI algorithms